Effectiveness of Mental Health Care by Using Machine Learning on Manufacturing Worker
Article information
Abstract
Despite the remarkable advancements in technology that have accelerated and enhanced manufacturing processes, it remains crucial to acknowledge the ongoing significance of variables associated with human mental health. Since the mental health should be cared, the rapid advancement of machine learning techniques has paved the way for innovative applications in the field of mental health care. This paper aims to provide a comprehensive analysis of published studies that utilize machine learning for the diagnosis & detection and treatment & support of mental diseases. The analysis reveals that machine learning techniques have predominantly been used for diagnosing and detecting mental diseases, with a particular emphasis on conditions such as depression, Alzheimer’s disease, and schizophrenia. Moreover, analysis on diagnosis & detection by using machine learning was conducted with a particular focus on categorizing the studies into high and low accuracy based on a threshold of 80%. Moreover, the review highlights the importance of considering various machine learning methods, as well as the utilization of various data types. Nonetheless, the limited number of studies focusing on treatment and support signifies an area that requires further exploration. This review emphasizes the need to minimize variables that may occur in the manufacturing process through mental health care, and mental health care research using machine learning should continue.
1 Introduction
Advances in technology have made the manufacturing faster and more sophisticated. Although the manufacturing has become fast and sophisticated, the significance of human labor remains integral. Most of the variables that exist in the manufacturing process can be controlled, however, humans always have potential to generate variables in manufacturing. One of the potential variables that human generates can come from mental health. For example, a person under high stress may make inappropriate choices in an unexpected situation. Therefore, the management of mental health is significant both manufacturing and society. However, despite the importance of mental health care, they are not well treated [1]. A previous study conducted in the United States found that fewer than 50% of people who require treatment for mental disease receive care [2]. Although there are opportunities for treatment, the treatment of mental diseases is often avoided due to stigma, expense, privacy worries, and time restraints. Furthermore, a preference for taking care of problems on one’s own is also included, but is not limited to above reasons [3,4]. On the other hand, 123 million Americans do not even receive treatment because they live in federally designated Mental Health Professional Shortage Areas [5]. Additionally, 41% of individuals with severe mental disease and 62% of people with mental disease in general did not obtain any mental health care, according to the previous study [6]. Patients who want to recover from mental diseases sometimes discontinue treatment because of inadequate medication dosage in primary care and insufficient treatment settings in follow-up care [7].
Despite the lack of treatment efforts, the mental diseases can have a serious impact on manufacturing process, individuals as well as the whole society, diagnosis and intervention of mental diseases at an early stage is essential [8]. However, the traditional methods of mental health care are labor-intensive and time consuming due to face-to-face interviews, self-reporting, or questionnaire distribution [9]. Along with the inconvenient traditional methods and technological advancements, mental health care is improving due to utilizing digital technology. Digital mental health care refers to the use of digital technologies such as smartphones, apps, websites, and other online platforms to support the prevention, diagnosis, treatment, and management of mental health conditions. For example, the digital mental health interventions (DMHIs) have been developed over the past 25 years [10]. The traditional face-to-face therapy for mental disease has changed by therapy delivered via online or mobile platforms. Since the physical distancing protocols was enacted during the COVID-19 pandemic, DMHIs can be quickly adopted by healthcare providers as well as patients [11,12]. Furthermore, based on the possibility of expanding the accessibility of mental health care through DMHIs, there are many studies for digital health care and summarized in Lattie et al. (2022) [10]. Furthermore, the diagnosis and treatment of mental diseases using machine learning (ML) and big data science have also become increasingly popular in recent years. Overall, the goal of this review is to give a brief overview of how ML and data science have been used in mental health care, thereby providing insights for those who want to conduct research on the use of big data and ML techniques in mental health care. Additionally, the review covers the drawbacks of utilizing ML techniques in mental health care, while also exploring potential opportunities.
2 Mental Health Care by Using ML
Despite the various fields of mental health care existing, we focused on diagnosis & detection (n = 105) and treatment & support (n = 51) of mental disease by using ML techniques. The studies about diagnosis and detection of mental disease aimed to determine or assess the mental health state of an individual. Furthermore, investigations in the realm of mental illness treatment and support have incorporated machine learning techniques to prognosticate the onset of mental health conditions and scrutinize potential treatment plans and support among affected individuals.
Although there are many mental diseases, this paper provides a comprehensive summary of instances where ML has been used in mental healthcare, specifically focusing on 11 distinct diseases. The 11 distinct diseases, ranging from Alzheimer’s to stress, are listed in the y axis of Fig. 1. Moreover, Fig. 1. also includes the count of studies conducted for each respective disease in diagnosis & detection and treatment & support. The practical application of ML models in mental disease assessment relies on the factor of accuracy, as precise diagnosis and detection are crucial. Therefore, studies employing ML for mental diseases diagnosis and detection have been categorized based on their accuracy, with a threshold of 80% (or Area Under the Curve (AUC) = 0.8).
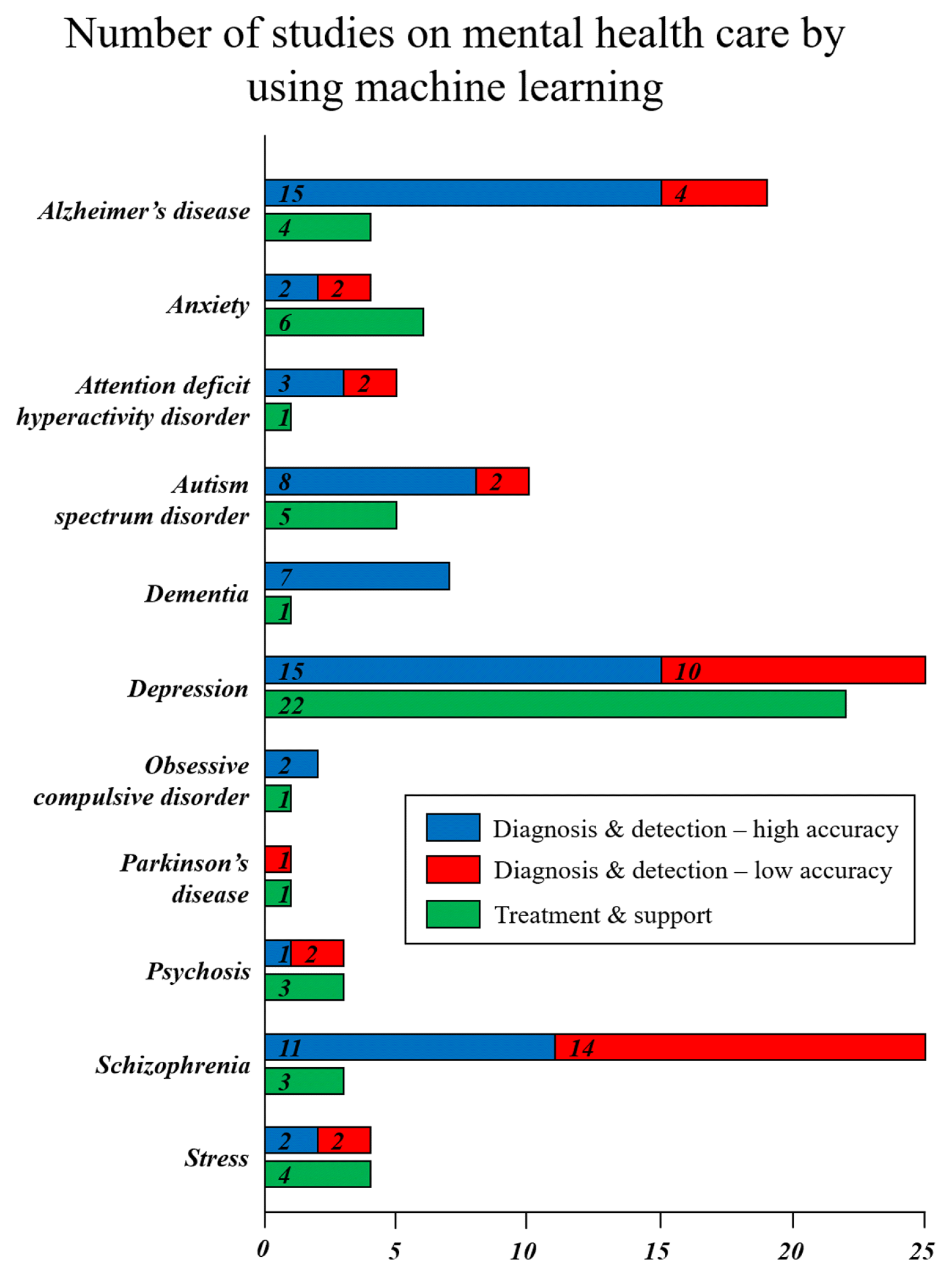
The number of studies conducted for each mental disease in the diagnosis & detection and treatment & support domains by using ML. Specifically, diagnosis & detection domain categorized by high or low accuracy with a threshold of 80% (or AUC = 0.8)
2.1 Diagnosis & Detection of Mental Disease by Using ML
The analysis presented in Fig. 1. reveals a predominant utilization of ML techniques in the diagnosis and detection of mental diseases, mainly focusing on depression, schizophrenia, and Alzheimer's disease. Table 1 also serves as an informative resource by presenting a comprehensive breakdown of disease-specific detection accuracy and distinguishing between high and low categories. Moreover, the table concisely summarizes the diverse machine learning techniques and data types utilized for each disease, offering valuable insights into their diagnostic potential.

ML techniques and data types used for diagnosis & detection of mental diseases. A total 11 mental diseases were investigated, and the studies on the disease was divided into high and low accuracy based on the diagnosis or detection of 80%
In the context of an Alzheimer’s disease-specific study, the application of nine distinct machine learning techniques was observed, specifically within papers that reported diagnostic and detection accuracies surpassing the established threshold of 80%. Regression and support vector machines (SVM) emerged as the most frequently used ML techniques among the nine distinct. Although both regression and SVM are ML techniques in supervised learning, their fundamental distinctions lie in their objectives. Regression aims to estimate continuous values, while SVM specializes in the classification of data points into discrete categories. Hence, when dealing with continuous data types like audio, employing regression techniques proves to be more advantageous in the learning process.
With it being recognized as a straightforward technique for examining discrete data, König et al. (2015) successfully harnessed the potential of SVM by employing feature selection methods, thus demonstrating the effective utilization of audio data within the SVM techniques [24]. The audio data, used in König et al. (2015), of normal and Alzheimer’s disease subjects are shown in Figs. 2(a) and 2(b), respectively. According to previous studies, the ML model acquired the capability to discriminate Alzheimer’s disease with an accuracy of 87 ± 3% in both the normal group (n = 15) and the Alzheimer’s group (n = 26) by extracting discretized features from continuous audio data. Additionally, the predominant data type used for the diagnosis and detection of Alzheimer’ disease is imaging data. For example, one study concentrated on harnessing supervised machine learning techniques in conjunction with neuroimaging data to effectively discriminate between Alzheimer’s disease and normal aging, highlighting the potential of this approach in diagnostic applications [19]. For better understanding, neuroimaging data used in previous studies are shown in Fig. 2 (c). Moreover, the utilization of magnetic resonance imaging (MRI) data with supervised learning techniques has been instrumental in diagnosing patients afflicted with Alzheimer’s disease, allowing for to notable levels of accuracy and demonstrating the potential of MRI as a valuable neuroimaging modality in diagnostic procedures [21,29]. In the case of diagnosing or detecting mental diseases using MRI data, it is essential to obtain and secure the necessary MRI data as the primary step. Subsequently, a small portion of the acquired MRI data is set aside as test data, while the remaining data is utilized for training purposes. As the training data goes through the feature selection step, the most relevant and informative features are selected from the preprocessed data to be used as input for the ML models. The ML model was built using extracted feature data, and has a process of distinguishing between normal and abnormal subjects. The ML model that has been classified is evaluated by the test data, and the aforementioned process is repeated until it obtains a satisfactory accuracy. The flow chart of the ML model is shown in Fig. 3. By following these flow charts, it becomes possible to classify Alzheimer’s disease with a high level of accuracy.

Examples of various data types used in ML techniques in order to distinguish mental disease. (a) Example of audio data of a normal subject. (b) Example of audio data of Alzheimer’s disease subject. (c) Example of the neuroimaging data
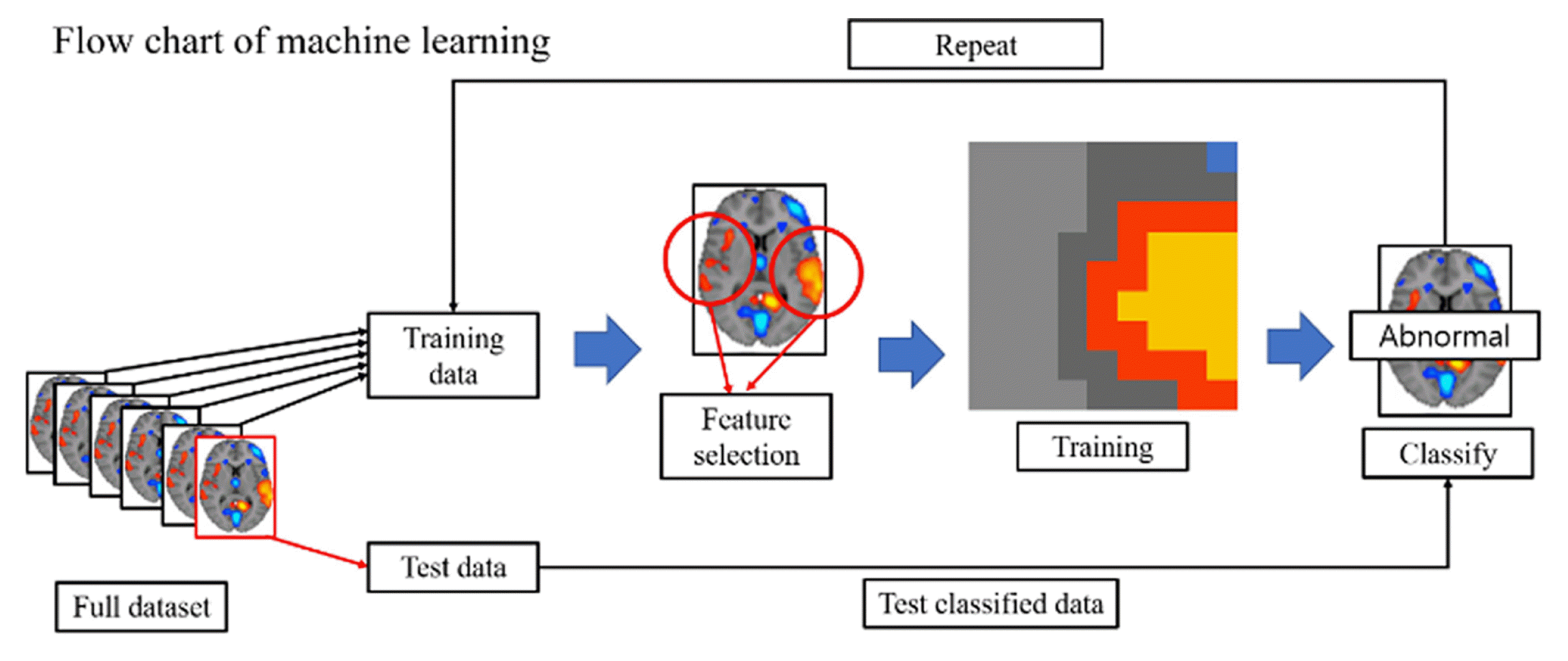
Schematic representation of the ML workflow for analyzing MRI data in order to diagnose and detect Alzheimer’s disease
Additionally, studies that reported relatively lower accuracy (below 80% or AUC < 0.8) in their results also employed a range of ML techniques, including SVM, random forest (RF), neural networks (NN), and decision tree (DT). All of the aforementioned ML techniques, namely SVM, RF, NN, and DT are supervised learning methods. Indeed, the predominant data type utilized in these studies is imaging data.
Extensive studies have been conducted examining the application of ML techniques for the diagnosis and detection of depression as well. This analysis conducts a comprehensive examination of 25 papers and reveals that 15 of them reported high accuracy. However, in comparison to the analysis made on Alzheimer’s disease, which reported high accuracies in 15 out of 19 papers, the overall accuracy of the analyzed papers was relatively lower. In papers reporting high accuracy, a comprehensive set of 14 ML techniques was utilized, showing the breadth and diversity of approaches employed to achieve reliable and precise outcomes. Similar to the trends observed in Alzheimer’s disease studies, SVM emerged as the most frequently employed ML technique among the 14 utilized in papers reporting high accuracy. While the majority of ML techniques employed in the studies were categorized as supervised learning, it is important to note that unsupervised learning methods, specifically principal component analysis (PCA), clustering, and k-means clustering, were also diagnose to diagnosis and detect depression. Unsupervised learning stands as a distinct branch within the realm of machine learning, where the primary focus lies in unraveling patterns or structures inherent in the data, without relying on labeled examples.
Unlike supervised learning, which necessitates labeled data for training, unsupervised learning delves into the underlying structure of the data, aiming to identify relationships or similarities among data points through an exploratory analysis approach. Similar to research conducted on Alzheimer's disease, the predominant data type was imaging data. This notable similarity highlights the significance of utilizing imaging modalities in the field of mental disease diagnosis and detection, underscoring their substantial role in enhancing our understanding of various mental disorders. Furthermore, it was evident that survey and social media data, which enables the assessment of an individual’s current emotional state, was actively taken into consideration in the examined studies [53,54,56,58,68,73]. Among the studies that reported relatively low accuracy, a total of 7 distinct machine learning techniques were employed. Specifically, the most frequently utilized techniques were SVM and regression. The analysis revealed that imaging data emerged as the most commonly employed data type across the examined studies.
Transitioning from depression, an analysis of the included studies also shed light on the application of machine learning techniques in the context of schizophrenia. The field of schizophrenia research has garnered significant attention, as evidenced by the inclusion of a substantial number of studies in the analysis, totaling 25 in count. In contrast to the previously discussed mental diseases such as Alzheimer’s disease and depression, the analysis revealed a notable difference in the distribution of accuracy reports for schizophrenia. Specifically, there were comparatively fewer papers reporting high accuracy outcomes compared to papers reporting low accuracy in the diagnosis and detection of schizophrenia using ML techniques. This discrepancy highlights the unique challenges and complexities associated with diagnosing schizophrenia and underscores the need for further research and innovation to improve the accuracy and effectiveness of machine learning approaches in this domain. For instance, Tron et al. (2016) did not utilize imaging data, which is commonly employed for the diagnosis and detection of schizophrenia [94]. Instead, the previous study aimed to diagnose schizophrenia by analyzing the activity of 46 individual facial muscles. In addition, to enhance the accuracy of facial muscle activity recognition, Tron et al. (2016) used real-time 3D face tracking technology, which is less affected by variations in head pose and lighting conditions, and served as the input data for the ML model. The example of 3D face tracking video data is presented in Fig. 4. The video data for 3D face tracking was designated as action unit, and subsequent classification of the normal group and the schizophrenia group was carried out by evaluating the intensity and duration of these action units. This innovative approach enabled them to achieve a high level of accuracy (AUC = 0.8) in diagnosing schizophrenia.

Incorporating advanced 3D face tracking technology improved the precision of schizophrenia diagnosis by capturing subtle facial movements
Among the papers reporting high accuracy in the diagnosis and detection of schizophrenia, a total of 9 distinct ML techniques were used. SVM emerged again as the most frequently utilized technique among these ML techniques. Moreover, consistent with the overall trend observed across various mental diseases, the analysis revealed that imaging data was the most commonly utilized data type in the studies. In the subset of papers reporting low accuracy in the diagnosis and detection of schizophrenia, the analysis revealed that SVM is most frequently used ML techniques and that imaging data was the most commonly employed data type.
2.2 Treatment & Support of Mental Disease by Using ML
Table 1 shows a noticeable disparity in the distribution of studies between the treatment & support compared to the diagnosis & detection of mental disease. Furthermore, Table 2 provides a comprehensive summary of the ML techniques and data types employed in the examination of treatment & support for the 11 mental diseases under investigation. This table offers valuable insights into the diverse range of ML techniques used to explore potential interventions and support strategies for individuals affected by these mental health conditions.

ML techniques and data types used for treatment & support of mental diseases. A total 11 mental diseases were investigated
Among the studies exploring treatment and support, the application of ML emerged by using mobile and sensor data to detect behavioral changes indicative of mental health conditions [118,156]. For example, Salafi et al. (2015) employed wrist-mounted sensors to capture physiological signals such as heart rate, skin temperature, and galvanic skin response, enabling the quantification of stress levels [156]. The wearable sensor is represented in Fig. 5(a). The overall hardware design and flow diagram of the stress index generator are also shown in Fig. 5(b). The study successfully identified stress conditions with a high level of accuracy, specifically achieving a classification accuracy rate of 91.26%. Furthermore, the study revealed that stress management could be facilitated by offering a deep breathing guide feature accessible through a mobile application.

(a) The wearable sensor used by Salafi et al. (2015) to capture physiological signals including heart rate, skin temperature, and galvanic skin responses for quantifying stress levels. (b) The hardware design and flow diagram of the stress index generator
In addition, the use of ML to deliver personalized and timely treatment or interventions were also studied [69, 95,108]. Yang et al. (2017) implemented automated video-based analysis as a method for diagnosing depression [108]. The study was initiated by defining the region of interest as the face and proceeded to detect 2D facial landmarks. According to the 2D facial landmarks, image processing was conducted, and the study achieved real-time diagnosis and detection of depression with high accuracy. The 2D facial landmarks and the image processing procedure are represented in Figs. 6(a) and 6(b), respectively. By means of this study, facial features were extracted in real time video, enabling the classification of depression based on the facial features. Additionally, the study revealed that the inclusion of voice data resulted in improved accuracy for discriminating depression. Furthermore, the analysis of online support groups within mental health communities was also conducted [127,128,130,142]. These studies collectively illustrate the numerous advantages of employing ML techniques in the realm of treatment and support. Notably, ML has demonstrated promising outcomes by leveraging smart meter data and neural networks to identify depression or Alzheimer's disease-related changes in sleep behavior [118]. Additionally, wearable sensor data, encompassing metrics such as heart rate, galvanic skin response, and temperature, coupled with both supervised and unsupervised ML methods, have proven to be effective in predicting stress levels [156]. Moreover, supervised ML techniques have been employed in conjunction with mobile sensors and survey data to deliver personalized interventions for depression [108]. Furthermore, the utilization of supervised ML in online communities has yielded valuable benefits, including the matching of patients with suitable support groups and the automated moderation of constructive comments in autism support forums [66,128].
3 Discussion
The objective of this paper is to provide a comprehensive synthesis of the existing studies on the utilization of ML in the context of mental health care. By examining current research and practical applications, this paper aims to offer insights into the advancements and trends in the field. The applications of ML techniques in the field of mental health can be categorized into two main domains: detection & diagnosis, and treatment & support. This paper explores and analyzes the various ways in which ML is employed in these areas, providing a comprehensive overview of its applications in mental health.
While there has been considerable research interest and significant advancements in using ML for the diagnosis & detection of mental diseases, it is important to note that the field of treatment & support has received relatively less attention. Moreover, analysis of the reviewed studies indicates a prevalent utilization of supervised classification techniques in the field of mental health care. These techniques, which involve training ML models on labeled data, have been extensively employed to classify and identify patterns associated with mental health conditions. While supervised classification techniques have demonstrated significant efficacy, it is essential to explore and evaluate the potential benefits of other ML techniques to gain a comprehensive understanding of their applicability and effectiveness in mental health applications. Further research and experimentation with alternative ML techniques can provide valuable insights and expand the range of approaches available for mental health analysis and intervention.
A notable observation from the reviewed studies is the predominant utilization of imaging data in the field of mental health research. Imaging data, such as MRI, electroencephalography (EEG), etc., has emerged as a widely employed modality for capturing and analyzing brain structures and activities. Despite the prevalence of sophisticated imaging techniques and advanced ML algorithms, it is worth nothing that there exists relatively simple assessment (e.g., self-report questionnaires and clinical interviews) for the diagnosis of mental health conditions. While these tools may not possess the same level of complexity or technological advancement as ML models, they offer practical and cost-effective means for evaluating individuals’ mental health status. Moreover, the simplicity of these assessment tools allows for broader accessibility and ease of implementation in various healthcare settings, ensuring that mental health evaluations can be conducted efficiently and accurately across different populations. Therefore, while ML techniques hold promise for enhancing diagnostic capabilities, it is essential to acknowledge the continued relevance and utility of simpler assessment tools in mental health practice and research.
One significant challenge in applying ML techniques to mental health care is the lack of consistency in accuracy. Although many studies use SVM and imaging data to build ML models, the variance in accuracy is quite large. This lack of consistency in accuracy highlights the need for continued research and refinement of ML models in the field of mental health. It underscores the importance of exploring different ML techniques, optimizing feature selection and engineering, and integrating multiple data sources to enhance the accuracy and reliability of mental health predictions and diagnoses. Furthermore, it emphasizes the necessity of robust validation studies and the incorporation of domain expertise to ensure the generalizability and validity of ML models in real-world clinical settings.
In the context of manufacturing, prioritizing the mental health care of workers is paramount and deserves careful attention. Integrating machine learning techniques for managing and addressing the mental health needs of workers has the potential to revolutionize the field, leading to what can be termed as smart manufacturing. By harnessing the power of machine learning, manufacturing industries can proactively identify and address mental health challenges faced by workers, enabling the creation of supportive and conducive work environments. The implementation of machine learning-based mental health care approaches not only enhances the overall well-being and productivity of workers but also fosters a culture of care and resilience within the manufacturing sector. It paves the way for optimizing human resources and improving overall operational efficiency while simultaneously recognizing the significance of mental health in the workplace.
4 Conclusion
The growing interest in mental health has led to a surge in research studies focused on mental health care. Researchers and clinicians are actively investigating various aspects of mental health care, including diagnosis & detection and treatment & support. However, currently reported studies have limitations such as: (i) research focused on diagnosis & detection of mental diseases, (ii) simple assessment tools for diagnosis other than ML techniques, and (iii) lack of consistency in accuracy. Overcoming these limitations will enable ML to contribute to more accurate diagnoses, personalized interventions, and improved outcomes for individuals experiencing mental health conditions.
Acknowledgement(s)
This research was supported by the Korea Advanced Institute of Science and Technology (KAIST) Entrepreneurial Partnership Program. (N10230147). We also would like to thank Lindsay Suyoon Park and Chelsea Sujin Park in Menlo-Atherton High School and Irene Choi in Singapore American School for their assistance.
List of Symbols
DMHIs
Digital Mental Health Interventions
ML
Machine Learning
AUC
Area under the Curve
SVM
Support Vector Machines
MRI
Magnetic Resonance Imaging
RF
Random Forest
NN
Neural Networks
DT
Decision Tree
PCA
Principal Component Analysis
EEG
Electroencephalography
References
Biography
Jongwoo Lim is currently a Ph.D. candidate in mechanical engineering from Korea Advanced Institute of Science and Technology (KAIST). He received the M.S. and B.S. degrees in mechanical engineering from Incheon National University in 2022 and 2019, respectively. His research fields are acoustics, vibration and finite element model for engineering applications such as bone conduction sound, topology optimization, and mode analysis.
Suhan Lee is currently a Ph.D. candidate in mechanical engineering from Korea Advanced Institute of Science and Technology (KAIST). He received the M.S. and B.S. degrees in mechanical engineering from KAIST in 2020 and 2018, respectively. His research fields are MEMS fabrication, thin film analysis, electrochemical materials for energy applications such as solid oxide fuel cell, solid oxide electrolysis.
Jinhong Noh is currently a Ph.D. candidate in mechanical engineering from Korea Advanced Institute of Science and Technology (KAIST). He received the M.S. degree in mechanical engineering from KAIST in 2021 and the B.S. degree in mechanical engineering from Pusan National University in 2018. His research fields are nonlinear dynamics and nonlinear vibration for engineering applications such as vibration/shock isolation, energy harvesting, additive manufacturing, cochlear mechanics, sensor/actuator, and vehicle/flight dynamics.
Wonhee Lee is currently a Ph.D. candidate in mechanical engineering from Korea Advanced Institute of Science and Technology (KAIST). He received the M.S. and B.S. degrees in mechanical engineering from Korea University in 2019 and 2017, respectively. His research fields are additive manufacturing, finite element analysis, biomechanical engineering, and applied mechanics.
Pei-Chen Su got her PhD degree from Stanford University in Mechanical Engineering with minor in Electrical Engineering. She has been working on several interdisciplinary topics in the areas of thin films and coatings for energy devices, micro- and nanofabrications, fuel cells and hydrogen, 3D/4D Printing, machine learning for air quality and weather prediction, machine learning for material and structure design for 4D printing.
Yong-Jin Yoon obtained his Ph.D. degree in Mechanical Engineering from Stanford University. He also obtained his Ph.D. minor degree in Management Science and Engineering with two M.S. degrees from Mechanical Engineering and Electrical Engineering. With his multi-disciplinary professional expertise, he focuses on research of MEMS-Microfluidic-3D Printing-enabled functional structures (sensors and biomedical applications) and novel Additive Manufacturing Process. Dr. Yoon founded and incubated several tech-startups (biosensor and 3DP companies) and participated as an investor and founder.